Quantifind Leverages Global Name Models to Identify the Right Mike, Fast
Quantifind has developed a global name rarity model for 172 countries that reduces false positives in KYC and AML name searches.
Financial crimes investigators have all felt the pain of finding news about the wrong person: A suspicious transaction alert flags a customer, and negative news then shows hundreds of potential matches with the same name. Identifying the right entity is crucial to customer due diligence (CDD) and Know Your Customer (KYC), yet false positives often dominate and can lead to significant downstream manual investigations.
Take the name “Mike Hamilton.” In the United States alone, there are more than 3,000 people with that name. With all the possible variations and without additional information such as age, how can institutions quickly, reliably, and accurately find the right Mike among all the noise? To make the problem more interesting, what if the Mike in question were not living in the United States?
The Quantifind solution uses machine learning to determine global name rarity, which, in combination with fuzzy matching and contextual models, brings cutting-edge technology to the anti-money laundering (AML) and Bank Secrecy Act (BSA) workflow.
Identifying the Correct Individual
A constant challenge for AML investigations and CDD efforts is prioritizing risks among limited investigative resources. One machine learning tool used to aid such efforts is named entity extraction, which leverages context to correctly extract a person’s or organization’s name from an unstructured text document.
But adverse media solutions in AML using this tool are only useful if they can determine that the subject of an alert is, in fact, the same individual identified in a negative news article. While many adverse media providers do this determination manually, their accuracy is notoriously poor.
Using context and a deep set of training data, our model can use the name’s geographical distribution to better separate good matches from bad ones.
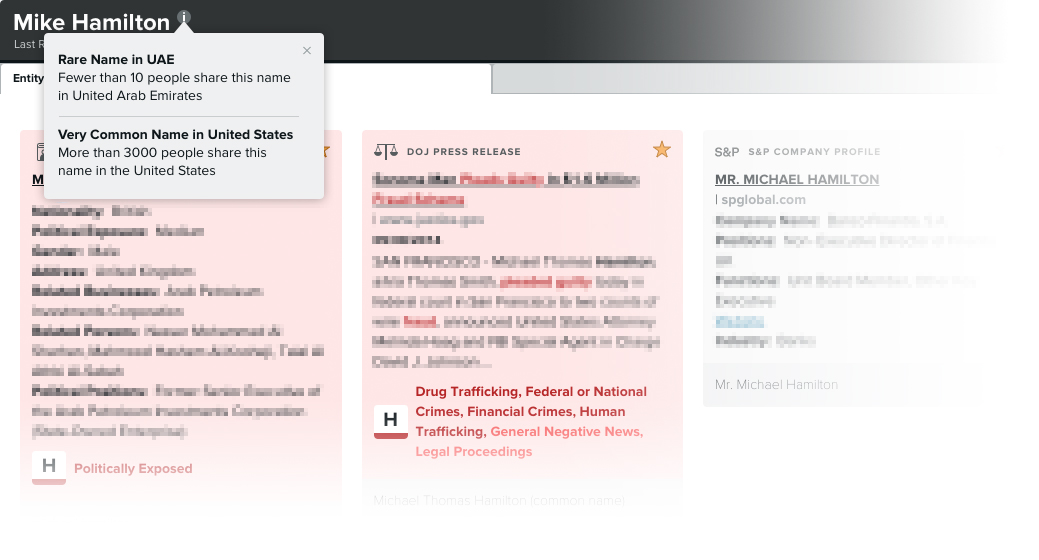
Scaling to a Global Solution
An article about Mike Hamilton residing in the United States, with no accompanying metadata, is unlikely to be referring to the same Michael Hamilton alerted in a transaction based on its common occurrence. However, if a Mike Hamilton were identified in UAE, in both an alert and a news article, the likelihood of them referring to the same individual increases significantly. Therefore, by using a negative news screening platform that determines name rarity by location, resources can be prioritized for the sources with the highest confidence.
Determining this name rarity is straightforward in the United States, as census data is easily available, and the sample sizes are statistically significant. However, this calculation gets harder when offering a global solution. While most financial institutions require global coverage, estimates of global name rarity are absent in most AML/BSA solution providers.
Quantifind, on the other hand, has built global name rarity models for 172 countries, by leveraging pairings of first names and surnames, with training data from more than 25 million samples. Our model accurately predicts name distributions in smaller countries by treating them as linear combinations of the distribution of names in the countries with sufficient data. Quantifind also uses deep learning techniques to deconstruct the structure of a name we haven’t seen before and determine its likely country of origin.
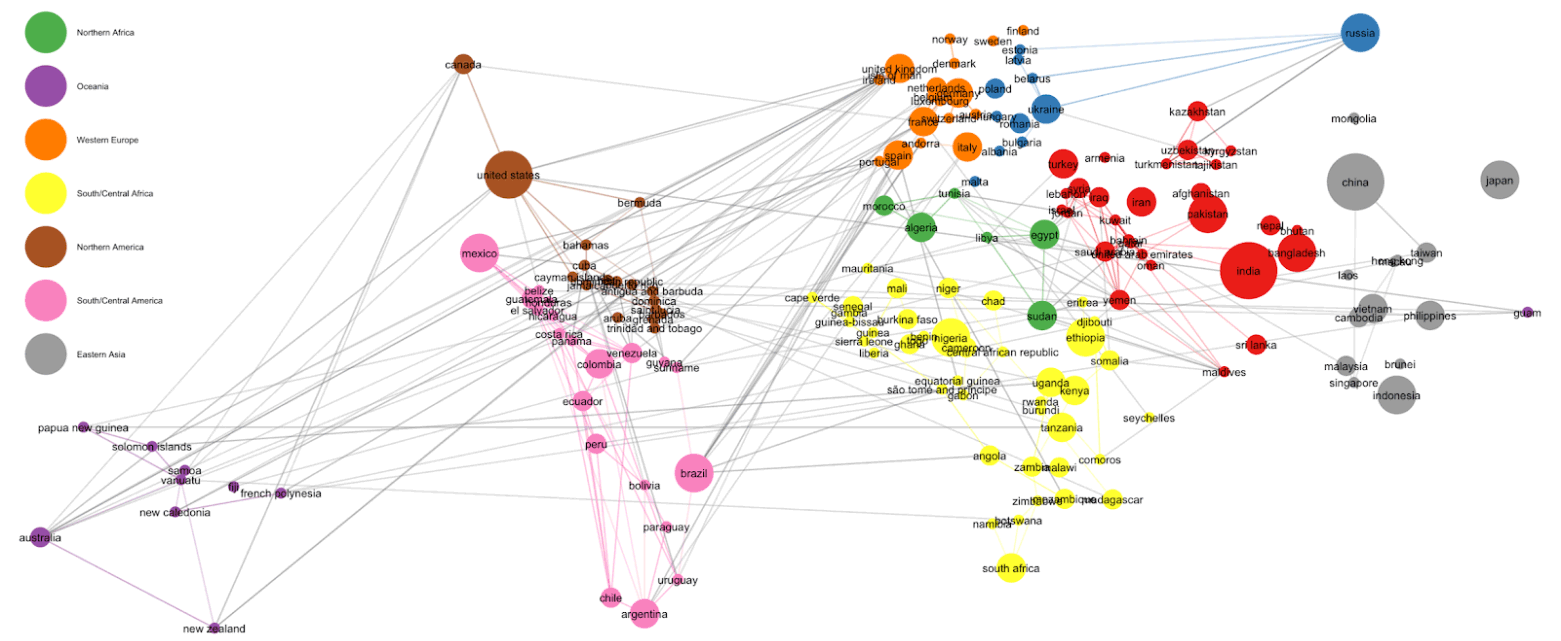
Our global name rarity model is just one aspect of our AI-driven solutions. At Quantifind, AI is not a buzzword, it’s how we achieve accuracy, relevance, and confidence in our results.
Using context and a deep set of training data, our model can use the name’s geographical distribution to better separate good matches from bad ones.